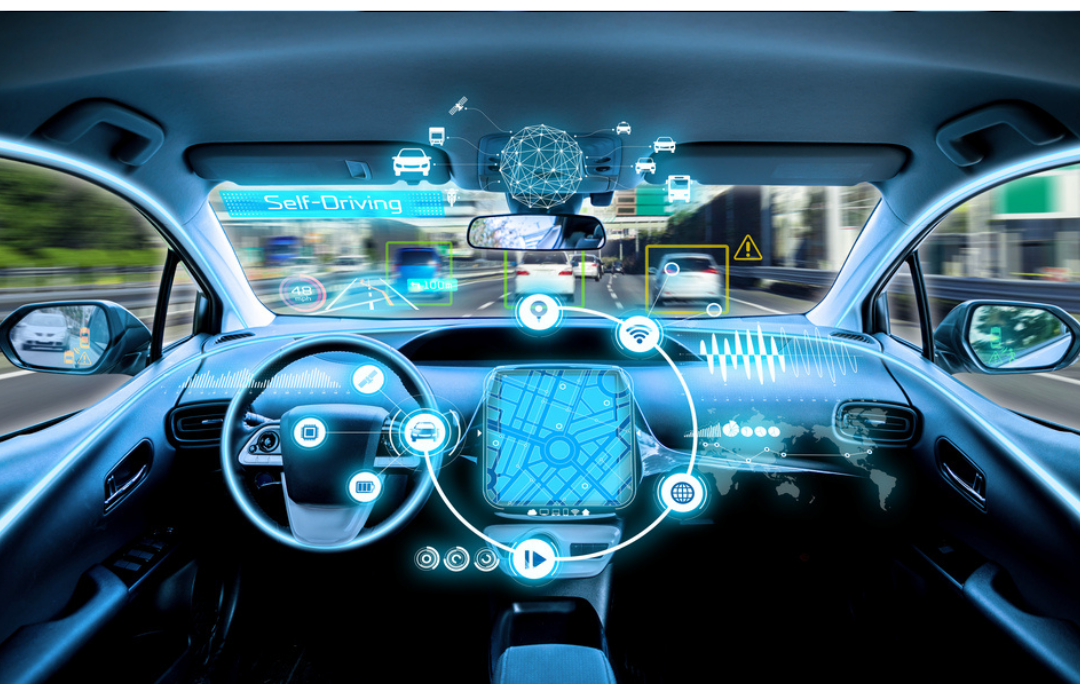
In 2017, Waymo CEO John Krafcik told tech conferences that “Fully self-driving cars are here.” The next year, however, at a Wall Street Journal panel with the title “Are we there yet?” Krafcik said that so-called “Level Five” self-driving cars were impossible:
“I’m not sure that we’re ever… going to achieve an L5 level of automation… I think it’s sort of silly that we think about it. And it’s important I think for all of us to be really clear on the language around self-driving because it does end up confusing people… autonomy I think is always going to have some constraint on it” [1].
Survey evidence suggests that consumers are indeed confused about whether they can currently buy a vehicle that is “self-driving” [2]. This muddle is not because the public are ignorant. It is because one of the major ways in which the development of self-driving cars has been discussed — the levels of automation drawn up by the Society of Automotive Engineers (SAE) — is misleading. A typology originally developed to provide some engineering clarity now benefits technology developers far more than it serves the public interest. We are social researchers who have over the last three years worked with and interviewed the developers of this technology. We argue that the levels of automation need a rethink. The SAE levels, by emphasizing autonomy and implying that progress means more autonomy, do little to inform public decision-making about the conditions in which these technologies might have meaningful benefits.
Self-driving cars could be a transformative technology in both good and bad ways. The important questions are not to do with when they will arrive but where, for whom, and in what forms they will appear. If we want a clearer sense of the possibilities from automated vehicle systems, we need to broaden our gaze [3]. Rather than emphasizing the autonomy of self-driving vehicles, we should instead be talking about their conditionality. We need to know about the circumstances in which different systems could have an impact on our lives. Self-driving vehicle systems will serve different purposes and take on different shapes in different places. A schema for innovation that points in one direction and says nothing about the desirability of the destination makes for a poor roadmap.
Where Did the SAE Levels Come From?
In order to represent and talk about new technologies, we need ways to describe them, compare them, categorize them, and keep in mind their risks and faults. As new waves of sociotechnical change have impacted daily life — electricity, radio, computerization — they have brought with them new terms. So it has been with automated vehicles.
As the terminology of automation has entered public and policy discourse, it has reinforced some myths of autonomy.
The taxonomy offered by SAE has provided a much-needed language by which to describe and compare the automation of driving. The six-rung ladder (Figure 1), ranging from Level Zero (no automation) to Level Five (full, unconditional automation) has enabled engineers to think about the technical differences between systems. However, as this terminology has entered public and policy discourse, it has served to reinforce some myths of autonomy: that automation increases linearly, directly displaces human work, and will continue until automation is total and humans are completely eliminated from the system [4]. The levels of automation have been treated as waymarks along that seemingly self-evident trajectory. It has become commonplace to describe a self-driving vehicle system as Level something, without further specificity. The Waymo
Chrysler Pacificas on public roads in Arizona and the low-speed Westfield automated shuttles operating with dedicated infrastructure away from public interference at London’s Heathrow Airport are both described as Level Four, but they have little in common. We need new ways to characterize such systems.
But first, a bit of history: In his account of the battle for automobile safety in the Twentieth Century, historian Lee Vinsel describes the SAE levels as an attempt at standardization [5]. The J3106 “Taxonomy and Definitions for Terms Related to Driving Automation Systems for On-Road Motor Vehicles” was first published by the Society of Automotive Engineers in 2014. By the time SAE published its standard, there were already two competing frameworks for increasingly automated vehicles. In 2013, the National Highway Traffic Safety Administration had drawn up five levels for self-driving vehicles. NHTSA had called Level Zero “no automation” and Level Four “full automation,” and focused primarily on the role of the human operator in carrying out “safety-critical control functions” [6].
An expert group of the German Federal Highway Research Institute (BASt) had in 2013 described its own levels for automated driving (as opposed to automated vehicles), from “driver only” to “full automation,” with the latter involving an automated detection of “system limits” and return to a minimal risk condition. In other words, there was no limitless “full autonomy,” in which the system could operate competently in any environment such as a licensed human driver can [7]. The members of the SAE’s task force, after reviewing the BASt document chose to largely adopt the BASt formulation with a few key changes [8].
The SAE made two interventions. First, they added a sixth level, “Level Five,” above BASt’s “Level Four.” SAE called their Level Four “High Automation,” and added” Full Automation” above it to disambiguate the conditions under which such a vehicle could operate. This also had the impact of dividing NHTSA’s Level Four, which described a vehicle capable of carrying out all safety-critical tasks without oversight, in two. In an SAE Level Four system, the entire driving task is carried out autonomously, but with some limitations on the environment in which the vehicle is expected to operate. These limitations are not specified by the taxonomy, but include such things as geofencing, controlled infrastructure, or even weather-dependent operation. A Level Five system by contrast is expected to perform under “all roadway and environmental conditions that can be managed by a human driver” [9].
Second, the SAE added more definitions and explanatory content. They were careful to explain that it is the task of driving, not the vehicle itself, that is being automated. They applied a precise definition of the driving task, which involved longitudinal and lateral control functions, as well as monitoring of the environment and fallback performance, although they equivocated on the “minimal risk condition” to which systems would be expected to retreat in the event of failure. The SAE approach gave the levels a technologically-centered, and less ambiguous, set of descriptions than NHTSA had provided.
The SAE levels have not been a static document, though the overall structure of the levels has not changed substantially. Through two revisions, in 2016 and 2018, the document has tripled in length, integrated more descriptive examples, especially around ambiguities and edge cases in the levels, and switched to increasingly specific, technical language to describe all aspects of the taxonomy. “System capability,” which described which “driving modes” could be handled by a system at a given level, has been replaced with “Operational Design Domain” (ODD), but otherwise responds to the same question: under what conditions can the system operate?
Implying that progress means more autonomy does little to inform public decision-making about how automation provides meaningful benefits.
Looking further back, frameworks for levels of automation in transport did not begin with NHTSA, SAE, or BASt. Some of the first examples of the modern, numbered levels format come from the late 1990s, when Endsley and Kaber developed a ten-level model for automation roles that was intended “to be applicable to a wide array of dynamic process and automated system control domains, specifically advanced manufacturing, teleoperations, air traffic control, and aircraft piloting” [10]. The authors drew on earlier attempts, such as Thomas Sheridan’s work defining roles of humans and machines descriptively, rather than numerically. Sheridan proposed that machines extend, relieve, back-up, or replace; and human supervisors trust, command, plan, monitor, and intervene [11]. The original role of Endley’s numerical taxonomy was to provide an ordering between more human control on one side, and more computer control on the other. It was not intended to encourage others to aim only for high automation, but to think about the options for appropriate partitioning of tasks to achieve a more reliable and functional joint-human-machine system. In a recent commentary, Sheridan has clarified “the difficulty, even impossibility, of making level-of-automation taxonomies into readily useful tools for system design” [12].
What Are the Current Problems with SAE Levels?
Thinking about how the structure of standards contributes to their use is therefore crucial for making better policy decisions.
As the historian Lee Vinsel argues, standards are not just ways of classifying things. They are also attempts to shape the technological future [13]. Thinking about how the structure of our standards contributes to their use is therefore crucial for making better policy decisions. The SAE’s standard levels formulation has a number of major weaknesses:
- The levels’ structure supports myths of autonomy: that automation increases linearly, directly displaces human work, and that more automation is better.
- The levels do not adequately address possibilities for human-machine cooperation.
- The levels specifically avoid discussion of environment, infrastructure, and contexts of use, which are critical for the social impacts of automation.
- The levels thus also invite misuse, wherein whole systems are labeled with a level that only applies to part of their operation, or potential future operation.
For self-driving vehicles, a typology that was developed to consider the possibilities and limits of machines in automating the task of driving has been stretched. In 2012 a U.S. Defense Science Board report argued that levels formulations are “often incorrectly interpreted as implying that autonomy is simply a delegation of a complete task to a computer, that a vehicle operates at a single level of autonomy and that these levels are discrete and represent scaffolds of increasing difficulty” [14]. This same critique could be leveled today at journalists and tech developers as they invoke the SAE levels. Obfuscation and hype around the levels has, according to some, contributed to recent crashes involving vehicle automation [15]. But these abuses are a function of the SAE levels themselves.
By their own description, the SAE taskforce added to the BASt levels by describing “categorical distinctions that provide for a step-wise progression through the levels” [16]. From a technical perspective, this tries to make the levels mutually exclusive and collectively exhaustive. But it also contributes to attempts to use the levels as a hierarchy of value or difficulty. Level Three automation has often been talked about in a way that puts it below levels Four and Five both in terms of interest and technical difficulty — the discovery that level Three might be more difficult to engineer than level Four, due to human factors issues in monitoring and fallback performance, is commonly talked about as a surprise to researchers [17]. Level Five has often been treated as a self-evident final goal. The levels’ direction bias toward more autonomy drives a technical bias (toward more data, more sensors, more compute) while ignoring other technologies and possibilities that may be equally valuable for making vehicle automation systems work in practice. The perspective brings some innovations to the foreground, such as sensors and processing power, while others are pushed to the background, such as digital connections between vehicles, high-definition maps and smart infrastructure. Once we broaden our gaze beyond artificial intelligence, we can see that the most profound benefits of “autonomy” may paradoxically come with greater connectivity.
Recognizing the straining of the framework’s usage, the 2018 version of SAE J3016 has clarified that the levels are “nominal, rather than ordinal,” and do not claim to represent “merit, technical sophistication, or order of deployment” [18]. But this does not match people’s intuitions about numbered categories, and the levels continue to be invoked in ways that go against their stated purpose.
The fundamental problem with the SAE’s framework may be rooted in the same myths that structure popular discourse. As some human-robot interaction researchers have described levels of automation formulations:
“The problem with such approaches is their singular focus on managing human-machine work by varying which tasks are assigned to an agent or robot on the basis of some (usually context-free) assessment of its independent capabilities for executing that task” [19].
From this view, levels of automation rule out forms of cooperation between human and machine. SAE levels assume that the problem to be solved — the task to be automated — is well-understood. So the project becomes one of substitution of the driving task rather than positive transformation of mobility. The SAE approach also sets the terms for the governance debate. The relevant question is seen as one of responsibility (in the narrow sense of liability) for the car, not responsibility for future transport. For places like streets and activities like moving, which are necessarily interactive and collaborative, such an approach may prove counterproductive. Johnson and colleagues recommend approaches based on interdependence between human and machine agents rather than autonomy [20]. To the issue of cooperative driving, we might also add the need to consider cooperative approaches to transport planning and the need to negotiate the desirable uses of shared spaces like roads, rather than presume that the correct approach is one of technological disruption.
As an alternative to the car-centered paradigm, low-speed automated shuttles have begun to be tested in many places in conditions that are constrained, either by tight geofencing or with the modification of infrastructures to suit the technology. These shuttles are often referred to by their developers as “Level Four” vehicles, even though their evolution was very different from the modified cars that are now being tested on public roads. These vehicles never had a past life involving a human performing a “driving task”. As systems, their design is closer to longstanding driverless forms of transport — including light rail and subway systems — that no longer attract curiosity. They do not quite fit into the SAE taxonomy, but nevertheless represent real alternatives to automobile-based systems.
Historically speaking, levels formulations have been more successful in cases where the environment can be constrained. For passenger trains, many of which are now automated and some of which are driverless, there are four Grades of Automation [21]. But the presumption is that other parts of the system are closed. London’s Victoria Line has operated as an automated system since 1968. The Docklands Light Railway has had no onboard drivers since its launch in 1987. The automation of these systems depends, crucially, on tightly constrained operational design domains. Railway systems, which rely on very simple, deterministic automation, work because other agents know their capabilities and limits. But these environmental and social constraints are lumped into an unspecified ODD, and therefore deemphasized by the SAE levels. The ODD is only generally defined: as “Operating conditions under which a given driving automation system or feature thereof is specifically designed to function, including, but not limited to, environmental, geographical, and time-of-day restrictions, and/or the requisite presence or absence of certain traffic or roadway characteristics” [22]. This definition externalizes much of what is most important to the operation of real-world systems.
Level Five remains utopian — an ideal but unattainable end state. A car that can navigate the streets of Phoenix, Arizona, would be disabled by the complexity of Rome or New Delhi. In reality, all systems will need to operate within constraints. Self-driving vehicles will not just adapt to the world as it is; to operate effectively, the world around them will need to adapt too. Automated systems only make sense in the context of their constraints, which means that a category defining an unconditional system is nonsensical.
When companies promise “full autonomy,” the SAE levels offer little help in holding them to account for what the promise means.
Furthermore, the SAE levels are sometimes deployed to refer to types of software, types of journey, responsibilities of drivers, parts of journeys or particular test conditions as well as the driving task. The dominant use, however, is to define a type of vehicle system, rather than a particular state or operational mode in a particular context. In discussions with people working in the industry, vehicles are often described as “Level Four” when they are capable of operating this way under certain sets of conditions, but might also operate at other levels of automation under different conditions. Currently, so-called “Level Four” vehicles are often tested with a safety driver who maintains actual responsibility for the system. Vehicles advertised as “Level Four” are often not “eyes off,” as the SAE category would suggest. So when companies talk about achieving “full autonomy,” the SAE levels offer little help in holding them to account for what that promise actually means.
To address this common misuse the 2016 version of J3016 added that the levels are mutually-exclusive by “feature.” Each automated feature has only one designation; but an entire “system” may have features that operate at different levels. This theoretically clean distinction between vehicles, systems, and features is however often blurred in practice. And it does not address the miscategorization of many experimental vehicles: these vehicles may be aimed at Level Four driving in the future, but they can be no higher than Level Three in practice, by SAE definition, if a backup driver is necessary to maintain safe operation.
Moving from Levels of Automation to Conditions for Operation
The SAE levels, as originally developed and subsequently invoked, have contributed to a particular narrative of self-driving vehicles. From this view, there is a clear problem to be solved and a race to achieve the solution first. It’s a view that suits some technology developers, but does little to help societies make good decisions about technology. As Pittinsky suggests elsewhere in this issue, the responsible development of algorithmic technologies demands consultation with diverse perspectives [23]. Regulators, consumers, transport planners, and citizens need new ways to talk and think about the possibilities and limits of self-driving vehicles.
An innovation schema pointing one way, but saying nothing about the desirability of the destination, makes a poor roadmap.
First, new typologies should start with the recognition that, if they come from authoritative sources, they are devices for communication as well as analysis. There is therefore a responsibility to publicly clarify the limits as well as the possibilities of particular systems. This demands a greater focus on the operational design domain and varied options for human-machine collaboration and interaction, and a downplaying of autonomy and the direct replacement of human beings with machines. To the extent that the driving task is a focus of a new framework, it must be open to new arrangements of shared human-machine control and collaboration. And developers should avoid using a numbered levels structure that implicitly orders the categories in terms of difficulty and value. But if AVs are going to change the world, we also need to know more about technologies’ relationships with their contexts. Policymakers and the public need clearer information about the conditions in which particular automated devices can operate, and the additional changes that might be required in order for such systems to be safe, equitable, and effective. This means less focus on the “driving task” and more attention to place, infrastructure, and road rules.
On infrastructure, we might look to the Infrastructure Support levels for Automated Driving (ISAD) recently proposed by the INFRAMIX project [24], which seeks to categorize parts of roads according to their connectivity. To this, we could add consideration of physical as well as digital infrastructures. Material arrangements of roads and road furniture are harder to standardize than digital systems, and vary more from place to place. In addition to varying road types, places are defined by various cultures and patterns of road use, which might make some AV systems inadequate or wholly inappropriate.
Social factors on the roadway, neglected by the levels today, are therefore of similar importance to physical and digital infrastructure. The SAE levels have little to say about the behavior of other road users, but AV developers are now starting to admit that the success of their systems may depend upon more predictable patterns of behavior from cyclists, pedestrians, and others [25]. New typologies for AVs should therefore be explicit about what else is required for the systems to function as designed. The real benefits of “autonomous” vehicles will come when they are embedded in and able to work with whole systems, including other road users and physical and digital infrastructures. We need ways to evaluate such systems, and ask old but important technology assessment questions: Who pays? Who benefits? Who decides?
Finally, policymakers need clearer ways to talk about technologies being tested and technologies being deployed. The widespread use of safety drivers as a fallback for prototype self-driving cars may be necessary for their safe development, but it means that testing for Level Four is happening, in effect, at Level Three. This means that these vehicles potentially come with all of the hazards of mixed-mode operation including mode confusion, automation complacency and problems of handovers. Notional future readiness for Level Four operation should not be allowed to confuse or distract from the real problems and risks that arise in testing and development, especially since the levels do not represent linear increases in capability. New typologies should aim for clarity about the conditions for testing as well as the conditions of use, and ensure that developers are being realistic about what will be necessary for their systems to be safe and effective in reaching stated goals.
The SAE levels have served their purpose, but they now look inadequate to the task of informing future discussions. The SAE levels are directing innovation towards greater autonomy, which could miss some larger opportunities. The focus of automation discussions needs to turn outward, away from the narrow technical capabilities of a system measured against a known human task, and toward the environments and conditions that can make safer, fairer, more accessible mobility achievable.
Retrieved from here.